While Emilio says he’s in good health, he feels his sleep quality has degraded over the years: “I have been trying to understand why and what could I do to improve it. There is nothing better than waking up in the morning feeling fully recovered and energised!”
Bedroom eyes
The concept for the tracker came from reading The MagPi, specifically a nature-watching camera project that used the NoIR Camera Module.
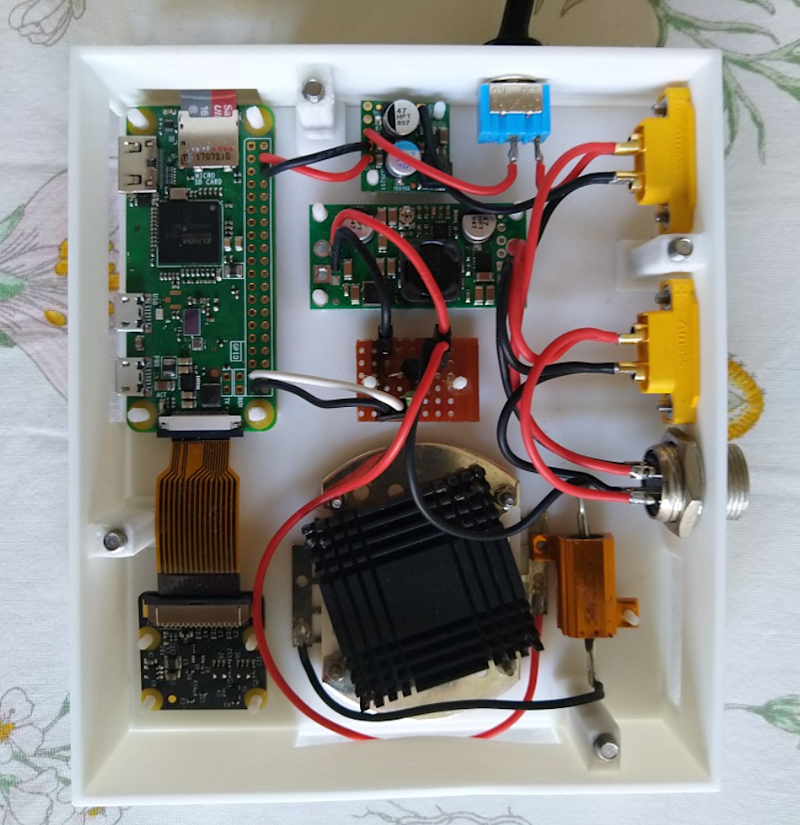
“I purchased a sleep tracker which provided me with very useful feedback,” Emilio explains. “However, sometimes the results from this device would not necessarily match how I was feeling in the morning. Some time afterwards, while reading in a TheMagPi issue a project where the NoIR Camera Module was used to take pictures of animals at night, I realised I could become one of those ‘animals’, and see if my own footage could help me understand those gaps.”
The software side used a series of images that Emilio had manually labelled as back, left, and right for the poses he was sleeping in. He then fed them into Google’s Teachable Machine to train the model to recognise the poses he wanted.
“This is a great way to very quickly sense how feasible the whole project would be,” Emilio says. “With 100 labelled samples for each class, and in less than ten minutes, I had a trained model downloaded in my local machine running inferences on the entire set of captured images.”
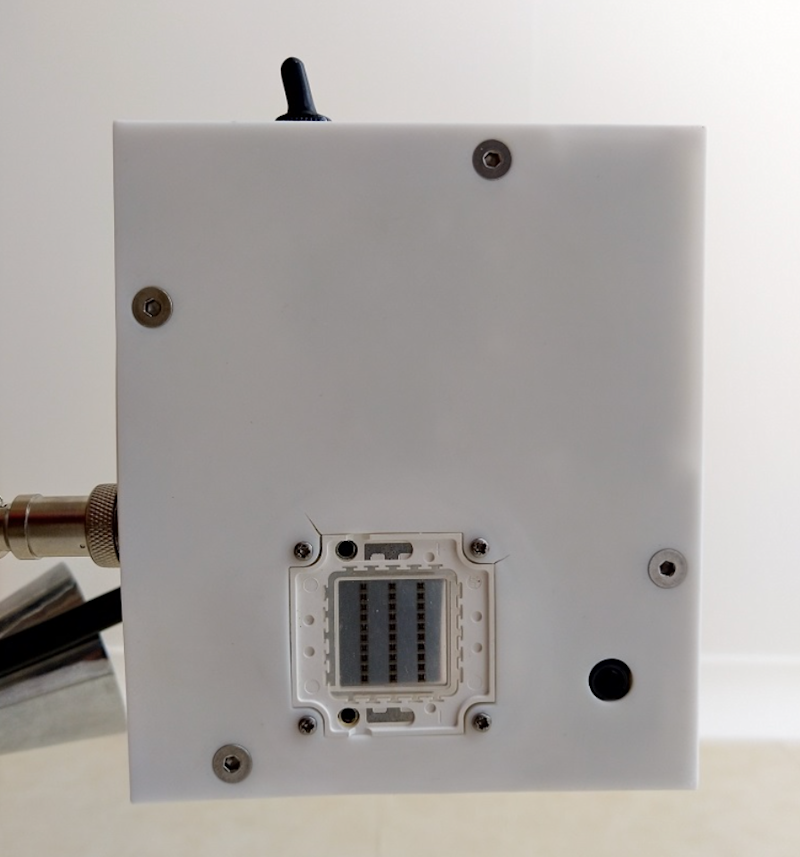
He then 3D-printed a cage for the components after some prototyping, including infrared LEDs to help with lighting. It’s powered from the mains, once it became clear that even beefy rechargeable batteries would not quite suffice.
Dream warrior
During sleep, a Python script runs over nine hours and takes one image every 15 seconds, which Emilio can then analyse.
“After manual inspection of all pictures over several nights, I can confirm the 15-second cadence is fit for purpose. I can even see some REM (rapid eye movement),” Emilio says. “So far, I only have some basic statistics from the last ten nights: on average I sleep 27% on my back, 41% on my left side, and 33% on my right side. Another interesting observation is that eyeballing the Oura ring sleep score against the percentages on each night, I can see that high scores tend to occur on nights with more ‘on right’ sleep.”
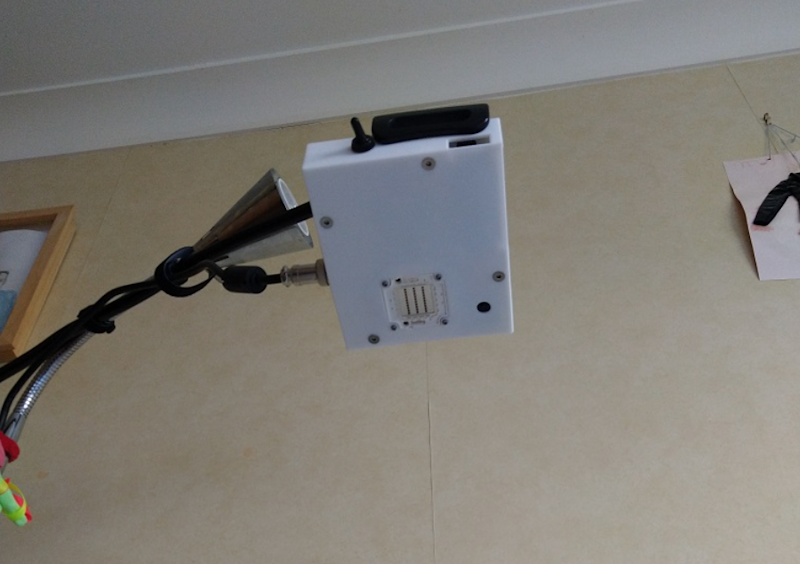
Emilio is continuing to work on the system, increasing accuracy, doing sleep research, and searching for patterns in his own sleep.
Quick facts:
Emilio ruled out medical issues like sleep apnoea
Identifying which side he was sleeping accurately took more training
Emilio plans to add more things for the system to measure
Real-time analysis using an Edge TPU will occur eventually
The limited LED use is so they don’t overheat